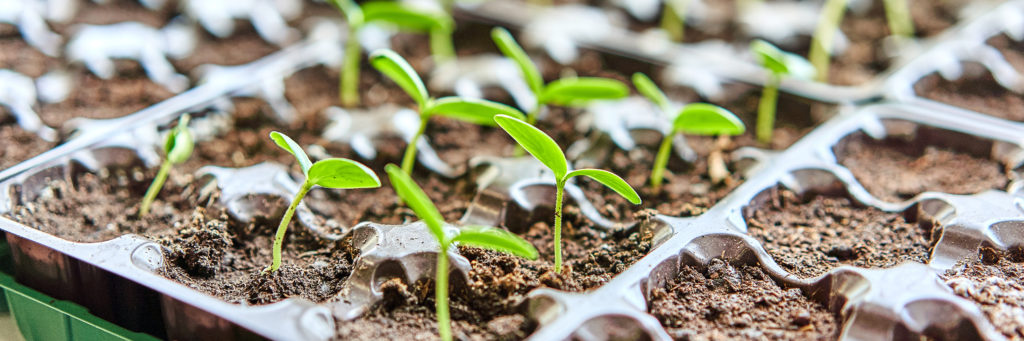
The Northeast Big Data Innovation Hub Seed Fund program is designed to promote collaboration and support the development and cross-pollination of tools, data, and ideas, leveraging data science innovations, across disciplines and sectors including academia, nonprofits, industry, government, and communities.
In 2020 and 2021, the NEBDHub awarded 25 Seed Fund grants from a pool of 72 proposals. Over $600,000 was granted to awardees across the northeastern U.S.
The projects were a great success, reflecting the following results:
- Education + Data Literacy (12 awards) was the most common focus area, followed by Health (10 awards), Responsible Data Science (7 awards), and Urban to Rural Communities (5 awards). Some awards spanned multiple Hub focus areas.
- 65 of the proposals were from academic institutions and 7 were from non-profits
- 23 of the seed fund grants were awarded to academic institutions and 2 to non-profits
- 5 of the grantees were from Minority Serving Institutions – 5 Asian American and Native American Pacific Islander-Serving schools and 3 Hispanic Serving Institutions.
- The broader impact of these awards was substantial, reaching over 36,000 individuals, including PIs, Co-PIs, graduate and undergraduate students, participants, and collaborators.
Read the below online booklet about the NEBDHub Seed Fund Success Stories, and meet the Seed Fund Steering Committee members from across the Northeast region who helped us bring this program to life.
Funding provided through this program is intended to support the northeast region and align with the Goals and Focus Areas of the Northeast Big Data Hub, as outlined on the NEBDHub About page. Click on one of the Four NEBDHub Focus Areas below for information on the NEBDHub Seed Fund Award Principal Investigators, Project Abstracts and Success Stories for that Focus Area.
Education + Data Literacy
All Aboard – Developing Protocols for Accessible AI Education
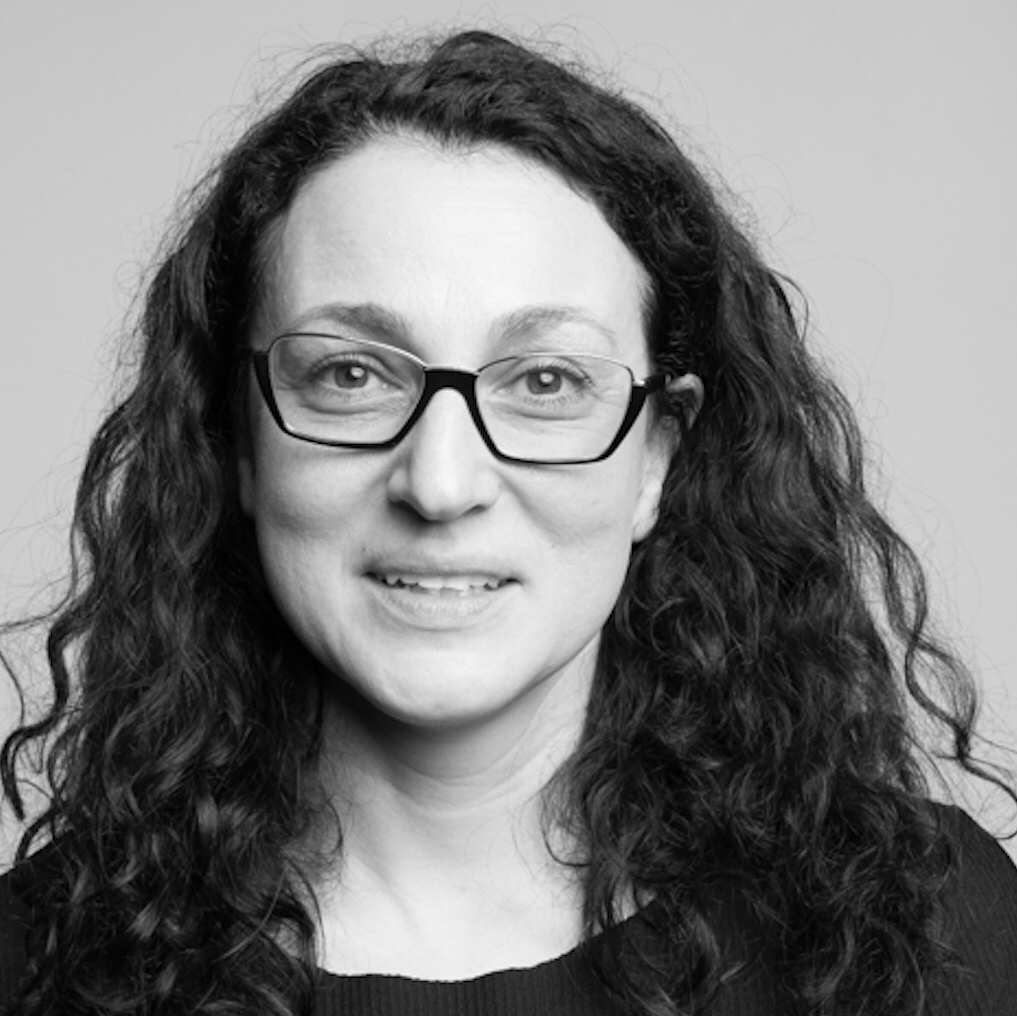
Lead PI
Dr. Julia Stoyanovich
(New York University)
Primary Focus Area
Education + Data Literacy
Secondary Focus Area
Responsible Data Science
Project Website
Project Abstract
As AI and data science applications take on significant roles in mediating our social lives, the democratic discourse around how these technologies should be developed, deployed, and regulated becomes increasingly important. Public education on AI and data science is central to this discourse. But this education – much as the technologies themselves – is not always equitable, especially when it comes to accessibility and participation from individuals with disabilities. The goal of the All Aboard project is to (1) convene gatherings, bringing together data scientists, disability scholars and activists; and (2) use the existing portfolio of NYU R/AI comics and public education videos as use cases to create a workflow for making public education efforts accessible. This project is a collaboration between NYU R/AI and the NYU Ability Project.
Expanding the Reach of DataJam: Introducing High School Data Science to More Diverse Youth, Communities and Regions
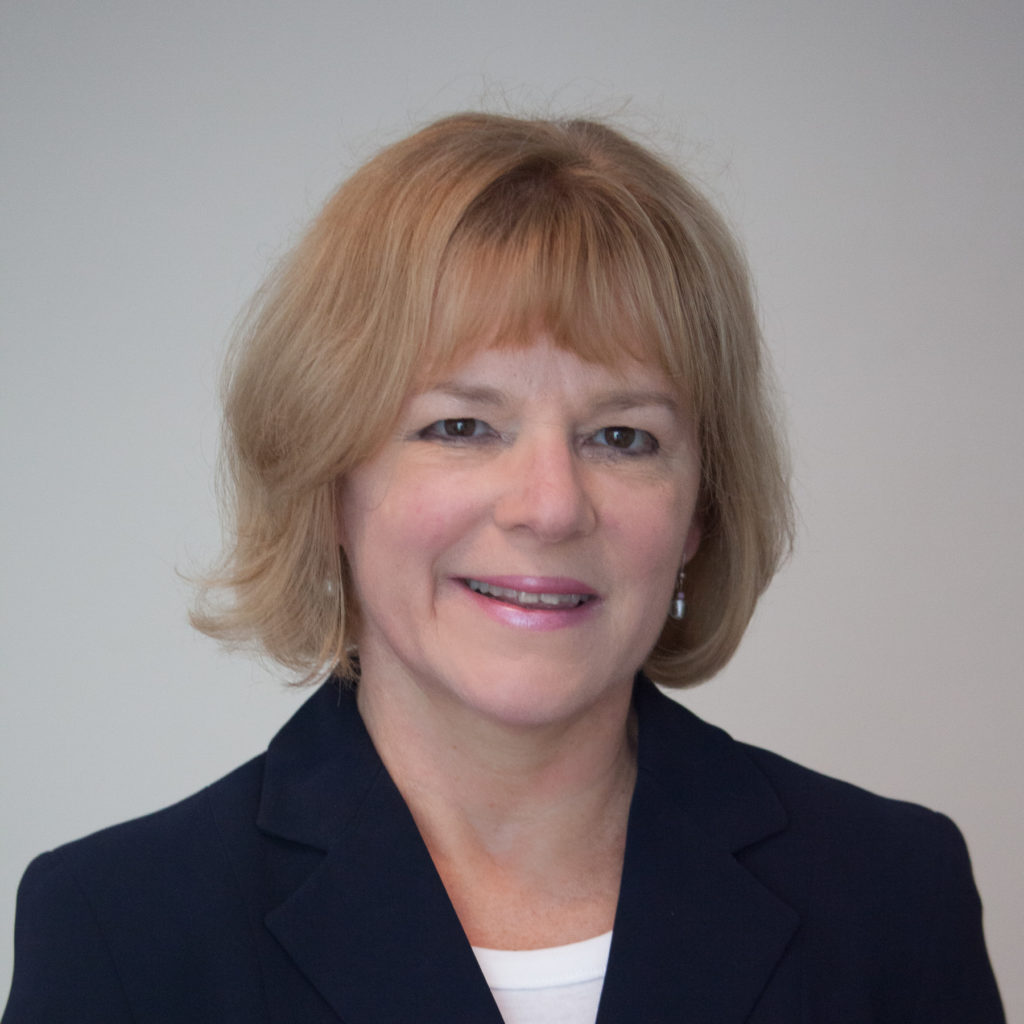
Lead PI
Dr. Judy Cameron
(Pittsburgh Data Works)
Primary Focus Area
Education + Data Literacy
Secondary Focus Area
Urban to Rural Communities
Project Website
Project Abstract
The DataJam is an academic competition, started in Pittsburgh PA 7 years ago, that runs throughout each school year and is designed to introduce high school students to the use of data to answer research questions. Teams of 5-7 students formulate a research question, find publicly available data sets, analyze their data, make data visualizations, and present their findings at a DataJam finale. Students learn skills pertaining to the scientific method, data analysis, and how to give scientific presentations. Teams are mentored by university undergraduate students who take a course on how to serve as effective DataJam mentors. In this pilot project, we will expand participation in the DataJam throughout the region served by the NE Big Data Innovation Hub through collaborations with groups well-connected to their local communities and high schools, with an emphasis on working with diverse learners from immigrant, rural, indigenous, and poor communities. This pilot project will specifically address 4 research questions: (1) What are the barriers or needs of communities of learners in engaging students in the Data Jam?, (2) What are the cultural assets or funds of knowledge that underrepresented learners and communities have that are relevant to undertaking a Data Jam project which will be engaging to them?, (3) What culturally contextual ways do students use tools and techniques to think with and communicate through data, and are they cross-culturally relevant?, and (4) Can we develop a hybrid model, a framework, for taking a strategy to teach data science to one population and effectively adapt it to work with culturally diverse populations of youth? We believe this knowledge is likely to be applicable not just to the DataJam but more generally to how to effectively adapt data science education strategies amongst diverse communities.
Teaching Responsible Data Science through Cybersecurity Analytics
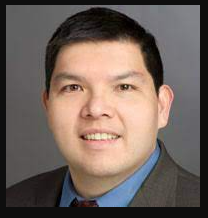
Lead PI
Dr. Shanchieh (Jay) Yang
(Rochester Institute of Technology)
Primary Focus Area
Education + Data Literacy
Secondary Focus Area
Responsible Data Science
PI Website
Project Abstract
The intersection of data science and cybersecurity is multi-faceted. Responsible data science requires sound measures and mindsets for security, privacy, and ethics. However, effective cybersecurity operations are in dire need of advances in data science and machine learning. In light of this intertwined relationship, we propose to cross-pollinate the teaching of responsible data science while simultaneously addressing problems in cybersecurity analytics. We will curate and homogenize cybersecurity data from open-source domains; produce a set of Machine Learning (ML) models that can be used in conjunction with the curated data to identify and predict cyberattacks; develop responsible data science exercises with the (ML) models; engage a broad range of communities, especially underrepresented groups as learners of the exercises; share the educational contents with video instructions through the Northeast Big Data Innovation Hub.
Curricular Structures to Blend Data Science & the Digital Humanities
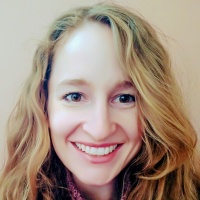
Lead PI
Dr. Amanda K. Greene
(Lehigh University)
Primary Focus Area
Education + Data Literacy
Secondary Focus Area
Responsible Data Science
Collaborators
Dominic DiFranzo (Lehigh University), Edward Whitley (Lehigh University), Annie Laurie Nichols (Saint Vincent College), Lauren Churilla (Saint Vincent College), Belle Lipton (Norman B. Leventhal Map & Education Center), Catherine Nikolovski (CIVIC Software Foundation)
Project Abstract
This seed grant will support a collaborative working group, bringing together academics and industry professionals to create shared pedagogical resources that integrate humanist perspectives, ethics, and data science. The group develop flexible, adaptable curriculum structures that support project-based learning and connect students to socially impactful data science projects beyond the classroom. By establishing learning outcome frameworks, creating model lesson plans with case studies and data examples, and running workshops during the grant term, this collaboration will drive innovative new digital humanities and data science programming at Lehigh University and Saint Vincent College. The resources that the working group develops will guide efforts to redesign existing courses, create new courses, and onboard new faculty for an innovative Digital Humanities major at Saint Vincent College and a Data Science + Digital Humanities certificate at Lehigh University. Emphasizing the role of data science in forwarding social justice initiatives and prioritizing ethical data literacy, these cutting edge programs and pedagogical structures will cultivate students’ technical capacities and enable them to apply socially conscious humanities skills in all phases of the data lifecycle.
Data Literacy as an Enabler to Broaden the Participation Of Underrepresented Minorities in STEM Careers
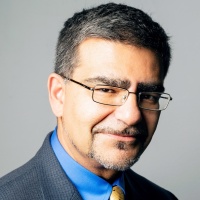
Lead PI
Dr. Babak D. Beheshti
(New York Institute of Technology)
Primary Focus Area
Education + Data Literacy
PI Website
Project Abstract
This project aims to increase data science capacity and talent, first by creating a sustainable pipeline from high schools and community colleges to universities for students to pursue degrees in computer science and data science, and second by increasing the accessibility of data science in the broader community. The objective is to expand data literacy and broaden the participation of underrepresented minorities and women in disciplines and ultimately careers in which an understanding of data science is foundational. The project’s goal is to make this course accessible to high school and community college students, as well as to the general public, by converting it to a fully asynchronous online mode. The project will also leverage partnerships with local high schools and community colleges to advertise and, through a competitive vetting process, provide scholarships for a group of students to take this course free of charge. This will allow students from lower-income communities and students underrepresented in data science fields to have access to these educational resources.
Building the Community to Address Data Integration of the Ecological Long Tail
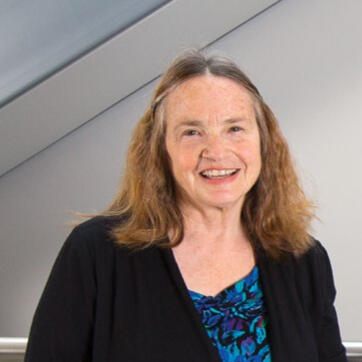
Lead PI
Dr. Beverly Woolf
(University of Massachusetts, Amherst)
Primary Focus Area
Education + Data Literacy
Collaborators
Ivon Arroyo (University of Massachusetts, Amherst), Will Lee (University of Massachusetts, Amherst), Danielle Allessio (University of Massachusetts, Amherst)
Project Abstract
This research focuses on Educational Data Mining, Learning Science, and Machine Learning. We build on current big data research and combine teacher inquiry and learning analytics to enhance teachers’ ability to collect and utilize real-time data about their students. The research will explore, evaluate, and apply machine learning techniques for optimizing and simplifying teachers’ assessment of students’ strengths, weaknesses, and socio-affective profiles to better create and adjust educational plans.
Development of a Data Analytics Learning Community
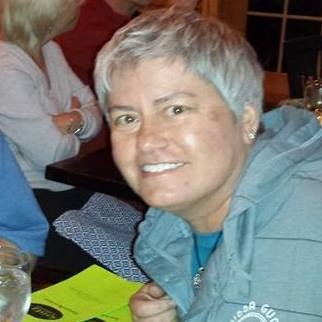
Lead PI
Dr. Cathie LeBlanc
(Plymouth State University)
Primary Focus Area
Education + Data Literacy
Collaborators
Daniel Lee (Plymouth State University), Rebecca Noel (Plymouth State University), Hyun Joong-Kim (Plymouth State University), Jonathan Couser (Plymouth State University)
Project Website
Project Abstract
The goal of this project is to increase the capacity of the existing faculty at PSU to teach data analytics, particularly in our General Education program. The project will result in a General Education course with data analytics content related to changing societal understandings of mental health team-taught by a data analytics expert and a historian. In addition, the project funds faculty participation in a data analytics learning community. The learning community kicks off with a week-long workshop in January 2021 through which participants will learn about data analytics as well as major principles in the science of learning and how those principles can be applied to the teaching of data analytics content. Each participant in the learning community has committed to incorporating some data analytics content into at least one of their classes. This project is seeding larger conversations on campus about the role that data analytics can play in student projects, particularly in our General Education program. Through our previous work with faculty learning communities, we know that discussions in these communities expand beyond those directly working in the community.
Building Tools and Training for Public & Educational Use of Geospatial Big Data
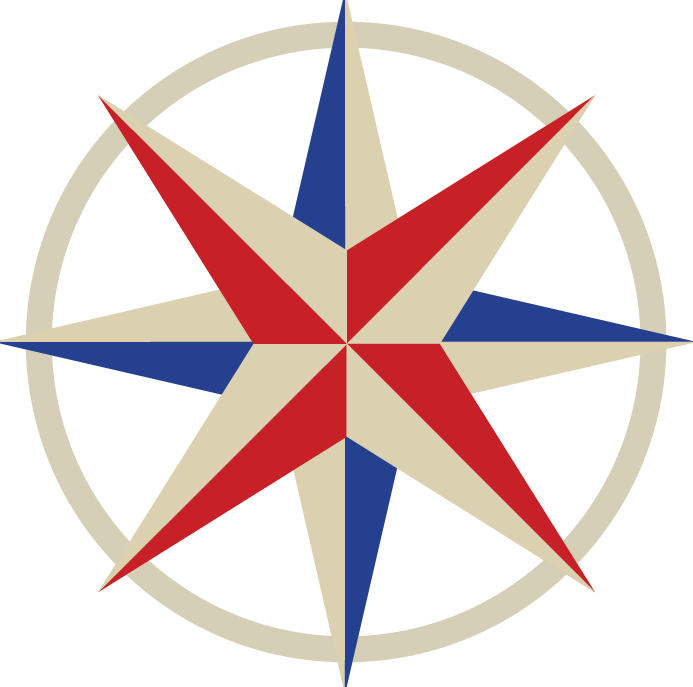
Lead PI
Dr. Garrett Dash Nelson
(Leventhal Map & Education Center at the Boston Public Library)
Primary Focus Area
Education + Data Literacy
Public Data Project
Leventhal Map & Education Center
Collaborators
Belle Lipton (Leventhal Map & Education Center at the Boston Public Library), Michelle LeBlanc (Leventhal Map & Education Center at the Boston Public Library)
Project Abstract
The Leventhal Map Education Center is a public-facing institution dedicated to geographic education using both historical materials and modern GIS tools. In this project, we will develop an interlocking set of “technical infrastructure” and “social infrastructure” aimed at equipping the public with better access to geospatial data, as well as better skills and critical attitudes in relationship to such forms of information. The technical infrastructure consists primarily of a bespoke data portal designed specifically for non-specialist users of geospatial data, incorporating human-readable metadata driven by concerns around data justice and data feminism. The social infrastructure consists of creating training materials for access to the public data portal aimed at K-12 public school teachers and library patrons, including both asynchronous tutorials as well as a multi-part introductory course, “Examining the World Through Maps of Data,” to be offered in spring 2021 as a free public program. Work will be supported by student interns associated with the MIT Data + Feminism Lab, as well as an advisory panel of K-12 teachers.
DEFLAB: Data Education and Feminism at Lafayette and Beyond
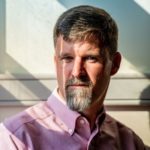
Lead PI
Dr. Trent Gaugler
(Lafayette College)
Primary Focus Area
Education + Data Literacy
Collaborators
Jason Simms (Lafayette College), Christopher Phillips (Lafayette College)
Project Abstract
Our project has three main goals. First, we will introduce local community college students to the fundamentals of data science through socially relevant projects. Second, we will enhance Lafayette College students’ ability to design data science projects and communicate data science methods to other students. Third, we will incorporate principles of “data feminism” into the entire project to facilitate students’ learning of data science with awareness of gender and other social inequities and strategies for promoting gender equity through the use of data—in other words, to show students that they can use data science to effect social change. By introducing college students to ways of understanding the role gender equity plays in data science and principles for promoting gender equity in their study and growth, this project offers a community-engaged, sustainable way to take steps from a world starting to reckon with the effects of its social ills to a world in which students well-prepared in both technical and liberal arts ways of thinking are equipped to take leading roles in working for the good of society.
Data Science Training and Research Program
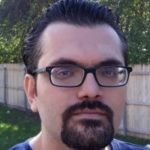
Lead PI
Dr. Yusuf Danisman
(Queensborough Community College, City University of New York)
Primary Focus Area
Education + Data Literacy
Project Abstract
This fully online program is aimed at enhancing the coding and data science skills of students at Queensborough Community College (QCC), a minority-serving institution located in Queens, NY. For this purpose, 10-15 students will be recruited for the Spring Semester of 2021. All students, as early as their first semester, can participate in the program. Underrepresented groups in STEM are especially encouraged to apply. This program will be organized into three modules: Python as a Programming Language (4 weeks), Data Science Machine Learning (8 weeks), and Capstone Group Project (3 weeks). During the program, students will attend virtual workshops and talks will be given by professionals in industry and academia. Students are also required to do weekly lab assignments and complete a capstone project as a group of 3-4 members.
Health
Nonlinear Dynamics and Machine Learning for Accurate Detection of
Early-stage Atrial Fibrillation
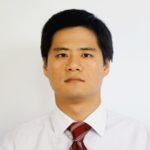
Lead PI
Dr. Changqing Cheng
(Binghamton University, State University of New York)
Primary Focus Area
Health
Secondary Focus Area
Education + Data Literacy
Project Abstract
The global coronavirus pandemic has put the once-niche telemedicine in the spotlight and is driving Health Internet of Things (HIOT) adoption in virtual health settings. Notably, the realization of the full potential of HIOT is highly dominated by novel data science tools that can transform the potentially elusive raw data into actionable information for timely detection and optimal intervention for chronic diseases, such as atrial fibrillation (AF). AF is the most prevalent abnormal cardiac rhythm with symptoms of rapid yet irregular heart beatings, and its early detection is paramount to reverse the course of progression and prevent further complications. In clinical settings, AF is often determined upon lengthy procedures, including inspection of symptoms (e.g., heart palpitations and chest pain) and physical examination (e.g., blood test and chest X-ray). Nonetheless, AF at the incipient stage is usually sporadic, and subtle symptoms can be fleeting or even absent. Hence, the in-the-hospital diagnosis procedure is not applicable for the early detection of arrhythmia. Therefore, there is a pressing need for an automatic and easy-to-deploy screening approach for early-stage AF in out-of-hospital scenarios. Whereas portable ECG-based monitoring abounds in literature, they mostly apply only to the long temporal data or rely on cumbersome data pre-processing and feature engineering in the time/frequency domain, or ignore nonlinear and nonstationary nonlinear dynamics underlying the short ECG. Another challenge resides in data imbalance: the majority of the ECG recordings are under normal conditions, whereas AF only represents a small portion. In addition, the ECG could also be contaminated by noise or artefacts due to the inappropriate contact between the electrode and the skin. All those factors have posed a quandary for accurate detection of early-stage AF and timely intervention with short-term ECG. The overarching goal of this proposed study is to develop an integrated platform to integrate nonlinear dynamics analysis and data science for incipient-stage AF detection. Specifically, the PI seeks to characterize short-term temporal data using nonlinear time series analysis techniques and tackle data imbalance via data augmentation with the generalized adversarial network. This will potentially add a new dimension to the evolving data science research and education.
A landscape of virus-host protein-protein interactions in SARS-CoV-2 infection in humans by machine learning
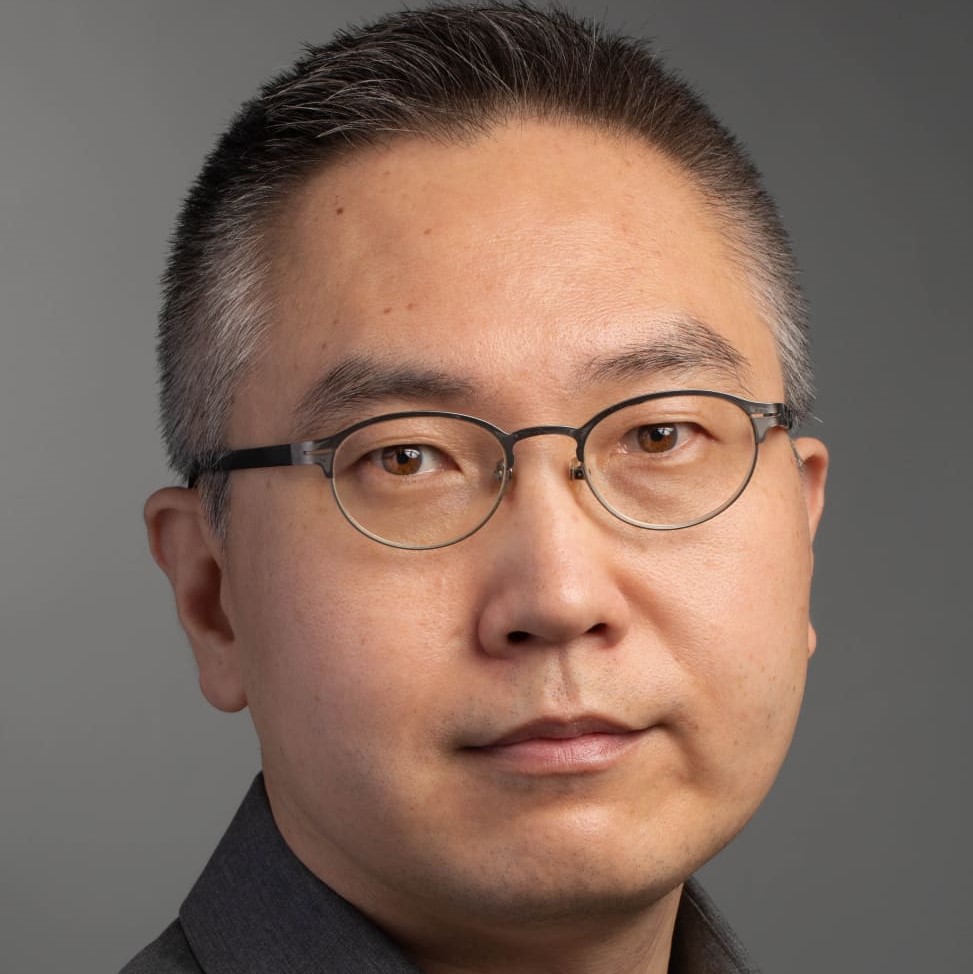
Collaborators
Prashant Emani, PhD (Yale University), Mark Gerstein, PhD (Yale University), Shrikant Mane, PhD (Yale University)
Project Abstract
We aim to predict and identify human proteins that are targeted by viral proteins of the novel coronavirus, SARS-CoV-2, that causes the COVID-19 disease, at the proteome level using advanced machine learning methods. We will use tree-based ensemble learning and deep learning models with protein sequence-based features as multi-class classifiers for multi-level evidence of virus-host protein-protein interactions. A large-scale public database will be used for model training and human target proteins predicted with high evidence will be functionally characterized for biological insight.
Convolutional Neural Network Facilitated Functional Cortical Mapping using tEEG Signals
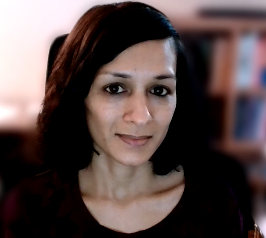
Lead PI
Dr. Kaushallya Adhikari
(University of Rhode Island)
Primary Focus Area
Health
Secondary Focus Area
Education + Data Literacy
Project Abstract
Approximately one per cent of the world population has epilepsy [WHO]. Many patients, children, in particular, cannot tolerate awake craniotomy and intraoperative language mapping, and this need drives the search for optimal preoperative noninvasive functional language mapping. Mapping of eloquent brain areas is critical for the preservation of function after respective brain surgery [Luders et al. Epileptic Disorders, 2006]. This creates a strong demand for reliable functional mapping methods in children, who are characteristically incompliant patients. The ideal pediatric paradigm therefore should be simple for the patient to perform and fast to acquire. A quick, high fidelity and noninvasive mapping technique are desired to address this need.
We proposed to perform functional cortical mapping using tripolar electroencephalography (tEEG) data. A convolutional neural network (CNN), a deep learning methodology, is a promising technique for tEEG data analysis. CNNs have been widely successful in computer vision, image processing, communications, and localization of neural dipole sources using EEG data. Since children cannot be expected to stay still for prolonged periods for tEEG data recordings, a robust methodology that can process data fast and reliably is a must for tEEG data processing and analysis. Moreover, since tEEG data have higher frequency signals than conventional EEG, they are recorded at higher sampling rates, typically 2000 samples per second or higher [Toole et al. Epilepsy u0026amp; Behavior, 2019]. Such high sampling rates can lead to voluminous data quickly, which calls for an automated and computationally efficient machine learning technique that has a potential for substantial speed-up and CNN is a promising candidate. We will perform a comprehensive statistical analysis of the sensitivity and accuracy of CNNs over different numbers of convolutional layers, input formulations, and activation functions. We will also compare the statistical analysis results with the outcomes of the minimum norm method, which is prevalent in EEG data analysis.
Contacts patterns during the 2020 COVID-19 epidemic
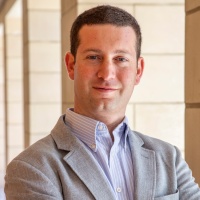
Lead PI
Dr. Eli Fenichel
(Yale University – School of the Environment)
Primary Focus Area
Health
Secondary Focus Area
Urban to Rural Communities
Collaborators
Anna Gilbert (Yale University), Roy Lederman (Yale University)
Project Abstract
Epidemics are social-behavioural phenomena. Smart device data are the key to understanding how human behaviors are changing during the COVID-19 pandemic. Our goal is to use individual-level smart device data to understand the behaviors driving the 2020 COVID-19 pandemic.
We acquired a unique data set of US smart device location data, where the average number of unique devices observed covers about 13% of the US population. These data pair two unique devices by hashed ID into co-locations. The data series runs from January 1st, 2020 through the present (and is updating daily with a 5-day lag). We also have a detailed spatial parcel and building data for the United States. We have merged the two data sets and propose to use the product to develop a platform for understanding the behavioral aspects of COVID-19 and specifically answering five questions:
1. What are the sources of COVID-19 infection? For example, are there specific contact patterns that are associated with hospitalization?
2. How do high-risk locations, e.g., meat packers, connect the broader community graph and spread infection?
3. How did, and are, individuals changing behavior in response to COVID-19 and policies?
4. What types of people should be prioritized for vaccination based on the contact patterns?
5. How can contact patterns be used to stratify COVID-19 testing surveillance regimes?
Using a data-driven approach to study health disparities and secular trends in the chemical and individual exposome in the NHANES
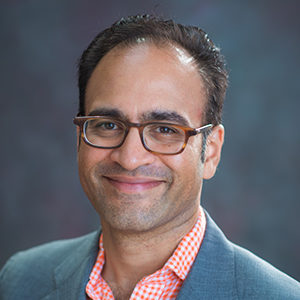
Lead PI
Dr. Chirag J Patel
(Harvard Medical School)
Primary Focus Area
Health
Secondary Focus Area
Education + Data Literacy
Collaborators
Ming Kei Chung (Harvard Medical School)
PI Website
Project Abstract
Health disparities remain a persistent public health challenge in the U.S. and disproportionate exposures to environmental hazards may be one of the major factors driving the disparities. For this project, we will systematically investigate chemical co-exposures in the U.S. to identify disparities in the patterns, correlations, and temporal trends of exposures in disadvantaged groups. We will leverage the rich chemical biomarker data available in multiple National Health and Nutrition Examination Surveys (NHANES). Specifically, we will divide our investigation into 3 specific aims: 1) estimating the temporal changes of the chemical mixtures in relation to the disadvantaged populations with simple and set-based statistics — Spearman’s rank correlation and canonical correlation analyses — and 2) calculating a novel “chemical Gini index” to succinctly quantify the environmental inequalities between and within the disadvantaged groups. 3) Using type 2 diabetes as an example, we will also construct a chemo-exposure risk score (CRS) to summarize the risks of developing diabetes from the totality of chemical exposures and investigate how CRSs and their temporal trends differ in the disadvantaged groups. Through this systematic study, we hope to prioritize mixtures of chemical exposures for actions – whether it is for research, policy formation, or chemical substitute development – and thereby helping to achieve environmental justice and reduce health disparities in the U.S.
A scalable computational pipeline to develop polygenic risk scores from biobank data

Lead PI
Dr. Hongyu Zhao
(Yale School of Public Health)
Primary Focus Area
Health
Collaborators
Robert Bjornson (Yale University), Wei Jiang (Yale School of Public Health)
Project Abstract
Genome-wide association studies (GWAS) have been very successful in delineating the genetic basis of human diseases. Tens of thousands of associations have been identified between single-nucleotide polymorphisms in the human genome and hundreds of complex traits/diseases. These GWAS results offer an opportunity to develop disease risk prediction models with genetic information and other risk factors, e.g. age and smoking. Because genetic factors make significant contributions to many diseases, accurate risk prediction using genetic information may significantly improve prevention, screening, diagnosis, and treatment for common diseases. These models derive polygenic risk scores (PRS) to quantify the genetic risk for a disease. The overarching goals of this project are to address the computational and implementation issues by developing a unified and user-friendly web platform for practising PRS analysis and benchmarking most existing PRS methods. More specifically, we will: significantly reduce the computational time of current PRS methods and integrate these methods within a toolkit with a unified input/output interface; build a user-friendly web platform for calculating genetic risks of many common diseases based on different PRS methods; and benchmark current PRS methods for different diseases, by using publicly accessible GWAS summary statistics from large consortia to develop PRS models and testing their performances by using more than 500,000 individuals from the UK Biobank.
CritCOVIDView: A Critical Care Visualization Tool for COVID-19
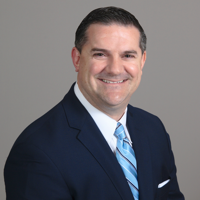
Lead PI
Dr. Todd Brothers
(University of Rhode Island- College of Pharmacy)
Primary Focus Area
Health
Collaborators
Abdullah Al-Mamun (West Virginia University)
Project Abstract
In the midst of the COVID-19 global pandemic, the US healthcare system is under exorbitant duress. As the mortality during the pandemic remains high, prompt decision making regarding medication use and treatment strategies requires overlapped insights of patient-centred data to be interpreted by the interdisciplinary critical care team comprised of pharmacists, nurses, respiratory therapists, and providers. Extracting and interpreting patient-specific health status data from multiple locations within the electronic medical records (EMR) is an arduous process and may lead to oversight of vital information. Currently, no tool exists visualizing the overlapped components of the EMR to support clinicians. The main goal of this project is to develop a cutting-edge tool titled CritCOVIDView to be utilized by bedside clinicians to interpret individualized patient data through the development of an interactive dashboard. Objective 1: Develop a data mining algorithm to understand the prescribed medication patterns and analyze the treatment modality complexities prior to and during the COVID-19 crisis. We will develop a custom association rule mining algorithm that will efficiently discover associations among the prescribed medications given the health status of critically ill patients. Objective 2. Develop an interactive critical care dashboard to visualize prescribed medications patterns, laboratory results, and vital signs to facilitate prompt decision making.
Harnessing Data to Predict and Prevent Cancer Treatment Adverse Events
through Artificial Intelligence
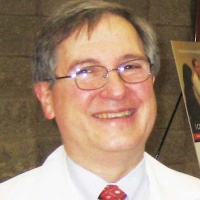
Lead PI
Dr. Robert Wieder
(Rutgers New Jersey Medical School, The State University of New Jersey)
Primary Focus Area
Health
Collaborators
Nabil Adam (Rutgers University)
Project Abstract
Adverse events from cancer therapy represent a significant challenge to patients’ well-being and survival and to the cancer treatment community. Adequate and rigorous approaches to predicting the occurrence of adverse events are lacking. Our long-term goals are to develop a systematic methodology to reliably predict and mitigate potential adverse events from cancer therapy. The specific aims of this proposal seek to understand the interactions of highly variable, exceptionally complex, multidimensional patient-, tumour- and treatment-related factors that predictably predispose to specific adverse events from breast cancer therapy. The approach will apply Artificial Intelligence (AI)/Machine Learning (ML) to the SEER/Medicare database to identify these interactions not previously recognized with existing approaches. Our novel approach will identify characteristics that collectively predict, with a high degree of confidence, the likelihood of adverse events in specific circumstances or at-risk subpopulations that can be ameliorated or avoided. The approach will permit intelligent hypothesis testing in prospective clinical trials to demonstrate the effects of modifying, avoiding, or ameliorating particular interactions of predictive variables to lower the probability or severity of adverse events. The results will provide contributions to the fields of Oncology, health care delivery, healthcare disparities, and big data.
Knowledge Graph Embedding Evolution for COVID-19
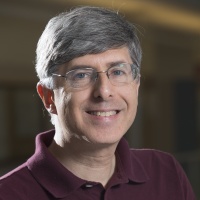
Lead PI
Dr. Steven Skiena
(Stony Brook University, SUNY)
Primary Focus Area
Health
Collaborators
Baojian Zhou (Stony Brook University, SUNY)
Project Abstract
In this seed fund grant, we propose to create and analyze a knowledge graph associated with COVID-19, studying how it has evolved in Wikipedia since January 2020. The COVID-19 graph presents a unique opportunity to study the development of a new topic from day 1. The evolution of graphs under models like preferential attachment proves successful at capturing power-law behavior, but does not adequately capture the evolution of knowledge growth reflected by graph and word embeddings of real-world networks. Our proposed work is expected to advance embedding models for these real-world networks.
Home-Bias as A Double-Edged Sword? Existence and Influence of Patients’ Preference for Local Physicians on Virtual Health Platforms

Lead PI
Dr. Shuting (Ada) Wang
(Baruch College, City University of New York)
Primary Focus Area
Health
Secondary Focus Area
Urban to Rural Communities
PI Website
Project Abstract
Geographic barriers have been widely observed in traditional healthcare systems. People in medically disadvantaged areas (e.g. rural cities) often have to compromise on the quality and the selection of local providers, due to the shortage of top-quality specialists who are usually concentrated in more populated big cities. The rise of virtual health platforms naturally leads to a question of whether virtual health can address such geographic barriers in healthcare. Ideally, the answer should be “yes”, as the platforms’ digital format allows patients to electronically consult providers without physically visiting the sites, enlarging their reach to the best providers in any location. Yet, the answer could also be “no”, because the influence of geographic barriers may also expand to the online setting. Specifically, patients may be compelled to choose local providers even on virtual health platforms, if the widely documented issue of home bias (viz i.e., a phenomenon wherein individuals irrationally prefer to transact business with agents that are within the same geographic boundary such as the same city, rather than those from outside) also exists in this context. Our study aims to empirically explore whether home bias exists among patients and if existing, what are the subsequent consequences.
Responsible Data Science
Procurement Roundtables: Algorithmic Justice and Responsible AI
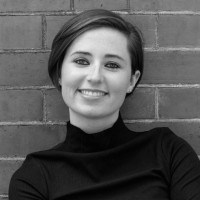
Lead PI
Dr. Mona Sloane
(New York University)
Collaborators
Rumman Chowdhury, Ph.D. (Parity), John C. Havens (IEEE)
Primary Focus Area
Responsible Data Science
Project Abstract
This project is a collaboration between the NYU Alliance for Public Interest Technology, the Institute of Electrical and Electronics Engineers (IEEE) and Parity, a collaborative platform that utilizes AI/ML to extract useful information from qualitative methods and combines them with rigorous quantitative assessments. It contributes to the emerging field of Public Interest Technology (PIT) and addresses the fact that there is little research and interdisciplinary exchange on issues pertaining to data science, public procurement, and transparency and justice. This is a glaring gap: 12% of the global GDP is spent following procurement regulation (World Economic Forum, 2020), and procurement is a core mechanism through which algorithmic power is distributed in public institutions. To fill this gap, this project will be comprised of three interdisciplinary “Procurement Roundtables” – one focused on data science solutions used by public institutions, one focused on algorithmic justice and responsible AI, and one focused on governance innovation. These roundtables will bring together experts in data science, social science (particularly critical technology studies), and governance.
Improving Data Integrity Awareness in HPC Datasets using Sparsity Profiles
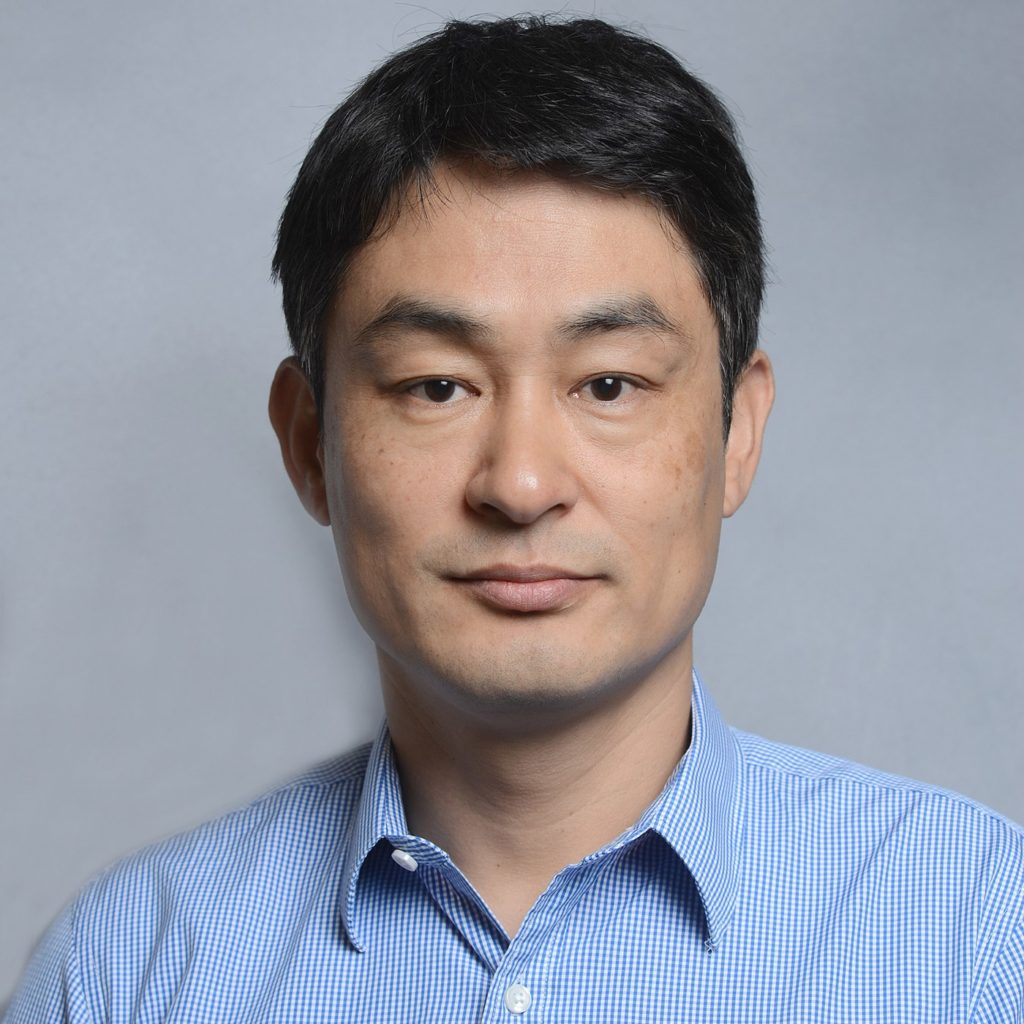
Lead PI
Dr. Seung Woo Son
(University of Massachusetts Lowell)
Primary Focus Area
Responsible Data Science
PI Website
Project Abstract
As the size and complexity of high-performance computing (HPC) systems keep growing, the scientists’ ability to trust the data produced is of the utmost importance. Of particular concern is data anomalies due to uncertain inputs, incomplete models, incorrect implementations, silent hardware errors, silent data corruption, malicious tampering, etc., which may stay undetected but cause wrong or incorrect answers. While machine learning-based anomaly detection techniques could improve the detection performance for HPC datasets, it is practically infeasible due to the increasing volume of data for inspecting anomalies, the lack of proper labels, and unwanted extra overhead associated. In this project, we exploit the existence of spatial sparsity profiles exhibited in HPC datasets for effective anomaly detection. The objectives are to apply compressive sampling to capture a compact representation of original datasets and build a model to classify normal or abnormal by analyzing the extracted sparse profiles. The proposed anomaly detection model will be evaluated against two popular unsupervised anomaly detection techniques with a range of contamination rates. It is anticipated that the proposed approach can detect point and collective anomalies with high accuracy while significantly reducing data size for inspection. This project leverages collaboration with computational scientists to cross-validate the proposed anomaly detection model in scientific applications and datasets.
Using Data Science to Study Environmental Racism, Justice, and Policy
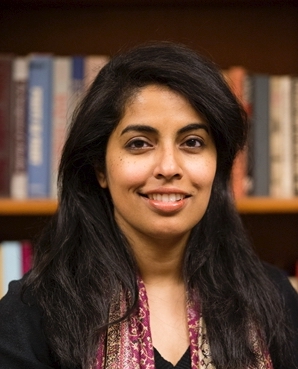
Lead PI
Dr. Aunshul Rege
(Temple University)
Primary Focus Area
Responsible Data Science
Project Abstract
Across the United States, thousands of families that reside in federally assisted housing are living on dangerously contaminated land where they face urgent ongoing environmental and health crises. In fact, 70% of hazardous waste sites officially listed on the National Priorities List (NPL) under the Comprehensive Environmental Response, Compensation, and Liability Act (CERCLA or Superfund) are located within one mile of federally assisted housing. That constitutes roughly 77,000 families living in public housing and homes paid for with vouchers at or near one of the nation’s polluted Superfund sites designated for cleanup by the federal government. These households disproportionately include low-income communities of colour. The proximity of contaminated sites to public housing is an example of environmental racism, instances of which range from the internationally criticized (climate change more acutely harming developing nations) to nationally condemned (lead-poisoned water in Flint, Mich.) to locally known (the legacy of waste treatment plants in the city of Chester). Access to affordable, healthy food is another matter of environmental justice. Many of the city’s community gardens are in the poorest neighbourhoods, under threat of development and pollution, which has changed the taste of residents’ food. This proposal offers a green criminology lens to the study of environmental racism and injustice using a qualitative data science approach to(i) create a harm matrix that focuses on impacts on the environment, health, and food, and categorize environmental injustice case studies using this matrix, (ii) compare the various case studies to identify patterns, rank the various harms along with incidence and severity, and identify corresponding remediation processes and costs, and policy recommendations, and (iii) verify the harms occurrences and prioritization rankings, any patterns, and response and recovery processes and costs by speaking with environmental and health subject matter experts.
Urban to Rural Communities
Forecasting Salinity in Rivers during Storm Events
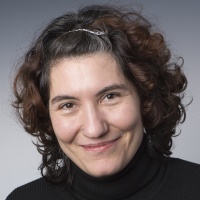
Lead PI
Dr. Laura Dietz
(University of New Hampshire)
Primary Focus Area
Urban to Rural Communities
Project Website
Project Abstract
This project takes a data science approach to forecast the salt concentration in rivers across New Hampshire. The purpose is to analyze what-if scenarios regarding salinity at particular river sites, in order to estimate the impact of changing weather patterns, such as rain-on-snow, drought, or intense rainfall, and different road treatment events.
Location-based Citizen Science in Augmented Reality Image Categorization
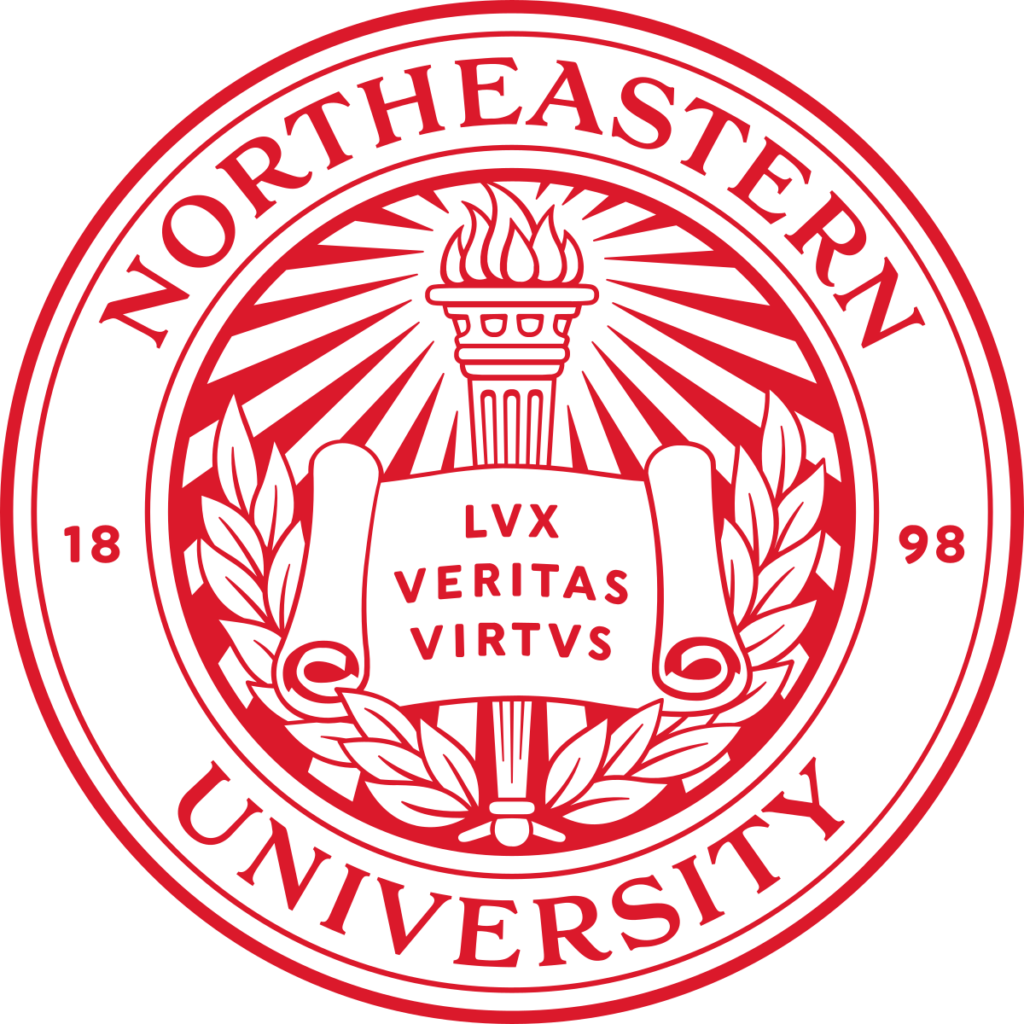
Lead PI
Dr. Seth Cooper
(Northeastern University)
Collaborators
Sara Wylie (Northeastern University)
Primary Focus Area
Urban to Rural Communities
Project Website
Project Abstract
Image categorization is a common citizen science task and can help to make sense of large image sets. The main goal of the proposed project is to integrate location-based images for categorization into the augmented reality (AR) citizen science toolkit we are developing. The toolkit, called Tile-o-scope AR, uses an AR mobile app to project images onto a set of physical tiles, which can be used for a variety of activities. The activities are built on matching images containing similar objects, which helps to categorize the images. In the proposed work we aim to add support for dynamically assembled location-based image sets, making the images to be categorized more relevant to participants. We will gather feedback from testers of our toolkit about their experience using location-based image sets.