Guest post by Dr. Seung Woo Son, Associate Professor, University of Massachusetts, Lowell
This Success Story is a report on the results of one of the awards in the Northeast Big Data Innovation Hub’s 2021 Seed Fund program.
As scientists conduct analyses that rely on large-scale simulations to achieve breakthroughs in many disciplines, their ability to trust the data produced is paramount. However, the data they generate, process, and transfer will be subjected to increasingly higher profiles due to various data anomalies, which may go undetected because of the lack of mechanisms to make scientists aware of data integrity compromises. The goal of this project was to exploit the existence of spatial sparsity profiles exhibited in scientific datasets for effective anomaly detection. A sparsity profile means that a few significant signal components could represent the given datasets concisely, thus minimizing the need for inspecting entire data points for anomaly detection. The University of Massachusetts, Lowell team produced an evaluation framework to inject errors in various data formats (binary, CSV, netCDF, etc.) using a diverse error injection metric (point vs. relative, gaussian vs. uniform). The developed framework has been open-sourced (https://github.com/swson/ADSP) and used for evaluating datasets for the PM2.5 prediction model. The Anomaly Detection with Sparsity Profile (ADSP) framework has also been used for evaluating various reference scientific datasets (https://sdrbench.github.io/).
As a result of this research, the team has published a short paper titled “Anomaly Detection in Scientific Datasets using Sparse Representation” as part of the Proceedings of the First Workshop on AI for Systems, held in August 2023. Authors of this paper include Aekyeung Moon, Minjun Kim, Jiaxi Chen, and Seung Woo Son. Additionally, a master’s student at the University of Massachusetts, Lowell was offered six credits of coursework for supporting this research project.
Additionally, these research findings were used to develop a grant proposal for NSF’s OAC (Office of Advanced Cyberinfrastructure) program in December 2022. The proposal, titled “Improving Data Integrity for HPC Datasets using Sparsity Profile” (NSF #2312982), was awarded in June 2023.
Lead PI: Seung Woo Son (University of Massachusetts, Lowell)
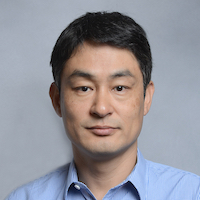
Seung Woo Son is an Associate professor in the Department of Electrical and Computer Engineering at the University of Massachusetts, Lowell. He was previously a postdoctoral researcher in the Electrical Engineering and Computer Science department at Northwestern University and the Math and Computer Science Division at Argonne National Laboratory. Son received his Ph. D degree in Computer Science and Engineering from The Pennsylvania State University in 2008. Prior to that, he was a research staff member at ETRI (Electronics and Telecommunication Research Institute), South Korea.