Guest post by Dr. Ho-Joon Lee, Yale School of Medicine
This Success Story is a report on the results of the Northeast Big Data Innovation Hub’s 2020 Seed Fund program.
COVID Information Commons Presentation: A landscape of virus-host protein-protein interactions in SARS-CoV-2 infection in humans by machine learning
Our goal with this Seed Fund project was to first build machine learning classifiers for multi-level evidence prediction of virus-human protein-protein interactions based on protein sequence profiles of interacting proteins. By applying those classifiers, we aimed to identify human proteins that are targeted by viral proteins of the novel coronavirus, SARS-CoV-2, that causes the COVID-19 disease, at the proteome level to offer insight into a SARS-CoV-2 interactome landscape.
We used tree-based ensemble learning models of random forests and XGBoost and deep learning models of GraphSAGE with protein sequence-based features for multi-level evidence prediction of virus-human protein-protein interactions. The large-scale public database of Viruses.STRING was used for model development. We achieved respectable performance of 74% AUC and 68% accuracy by the best XGBoost model. We made novel predictions of different evidence levels for SARS-CoV-2 virus-human protein-protein interactions in a comprehensive and unbiased way in silico, which could be considered as a new dataset of a virus-human protein-protein draft interactome. Human target proteins predicted with high evidence levels were also prioritized and functionally characterized for specific hypotheses, e.g. importance of cysteine and histidine in protein sequences and histone H2A as a target of multiple SARS-CoV-2 proteins.
As a result of this initial research, we are now developing an NIGMS Technology Development Program R21 proposal to the National Institutes of Health (NIH). The proposal is to develop a more general analytical framework for virus-host protein-protein interactions using various machine/deep learning models, and to integrate with our two other projects of drug repurposing and network controllability to target and disrupt those interactions.
The methods and predictions by random forests and XGBoost classifiers are published as a preprint in bioRxiv (https://www.biorxiv.org/content/10.1101/2021.11.07.467640). Results from GraphSAGE models will be incorporated in the next version.
Lead PI: Ho-Joon Lee
Collaborators: Prashant Emani, Mark Gerstein, Shrikant Mane
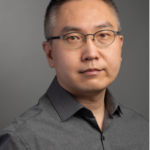
Ho-Joon Lee is an Associate Research Scientist at the Department of Genetics and Yale Center for Genome Analysis, Yale School of Medicine. He obtained a PhD in bioinformatics from Free University of Berlin and Max Planck Institute for Molecular Genetics in Germany and Master’s degrees in theoretical physics and applied mathematics from Cambridge University and Swansea University in the United Kingdom. His postdoctoral training was in systems biology at Harvard Medical School. His research topics include single cell biology, systems/network biology, and biomedical machine/deep learning. In response to the SARS-CoV-2 pandemic, he initiated a voluntary COVID HASTE working group at Yale School of Engineering & Applied Sciences (SEAS) together with Dr. Prashant Emani (Yale University) to study molecular mechanisms of SARS-CoV-2 infection and drug repurposing, part of which led to the funding from the Northeast Big Data Innovation Hub Seed Fund Program of 2020.
Collaborators
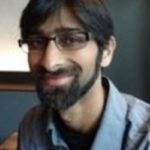
Prashant Emani, Associate Research Scientist, Department of Molecular Biophysics &
Biochemistry, Yale School of Medicine
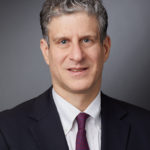
Mark Gerstein, Albert L Williams Professor of Biomedical Informatics and Professor of Molecular Biophysics & Biochemistry, of Computer Science, and of Statistics & Data Science
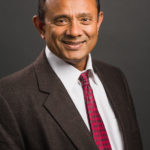
Shrikant Mane, Professor of Genetics; Director, MBB Keck Biotech laboratory; Director, Yale Center for Genome Analysis