Guest post by Dr. Kaushallya Adhikari, University of Rhode Island
This Success Story is a report on the results of the Northeast Big Data Innovation Hub’s 2020 Seed Fund program.
The goal for the project was to perform functional cortical mapping using tripolar electroencephalography (tEEG) and EEG data with convolutional neural networks (CNNs). The project used data from previous research efforts. To collect the data, the participants were shown a sequence of 50-drawings, each 3500 milliseconds long. The participants were shown a grey color image between 2 drawings for 2500 seconds. The participants identified each image under two conditions: overt (verbalize aloud the image name) and covert (silently name the image). Each condition was run twice (5 minutes long and total of 4 runs). During the experiment, their brain signal data was collected by tEEG and EEG, focusing on two important language areas in the brain, which are Broca and Wernicke.
This Northeast Big Data Innovation Hub Seed Fund project focused on classifying tEEG and EEG signals into right hemisphere and left hemisphere using CNNs. The researchers considered various input formats: spectrogram, raw two dimensional data, and two dimensional energy data. The results indicated that the CNNs cannot correctly classify left handed patients as right-hemisphere dominant and right-handed patients as left-hemisphere dominant based on tEEG and EEG signals.
The researchers also analyzed tEEG and EEG signals’ energy levels before and during language stimulation. After filtering the signals with a 60Hz notch filter (fs=2000Hz), the project compared the mean energy during the rest (or baseline) period with the mean energy during the stimulation period for different channels by using p-values test. We also analyzed the changes in the following frequencies: Delta (0.1-4Hz), Theta (4-8Hz), Alpha (8-13Hz), Beta (13-30Hz), and Gamma (30-100Hz). This analysis showed that the same subject can have different active brain frequencies and brain areas at different recording instants. These results were not identical for EEG and tEEG signals. Some right handed patients had active areas in the left hemisphere while other right-handed patients did not. For left-handed patients, either the right hemisphere or both hemispheres were active. For every part of the brain (as indicated by the electrode placement), there was always at least one active frequency content.
In November 2021, Adhikari’s team submitted a proposal to NSF Smart and Connected Health (SCH) based on this seed project.
Lead PI: Kaushallya (Kay) Adhikari
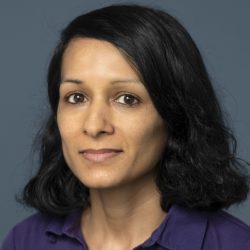
Kaushallya (Kay) Adhikari is an Assistant Professor of Electrical, Computer and Biomedical Engineering at the University of Rhode Island College of Engineering.