Guest post by Dr. Laura Dietz, University of New Hampshire
This Success Story is a report on the results of the Northeast Big Data Innovation Hub’s 2020 Seed Fund program.
Every winter, vast amounts of road salts are scattered onto streets across the northeastern U.S.. While important for our safety, ice-melt and rainfall wash this road salt into river systems where it damages our ecosystems. Often, the negative impacts of high salt concentrations in river systems only become evident after extended periods of time. For example, when Hurricane Sandy flooded New York City with salt water in 2015, the disastrous effects on trees became visible three years later.
So far, the transport of salt through our waterways is only poorly understood by biogeochemists and hydrologists. The “Forecasting Salinity in Rivers during Storm Events” project takes a data science approach in forecasting the salt concentration in rivers across New Hampshire. The purpose is to analyze ‘what-if’ scenarios regarding salinity at particular river sites in order to estimate the impact of changing weather patterns (such as rain-on-snow, drought, or intense rainfall) and different road treatment events. For example, if a dry period in winter is followed by multiple severe storms, would we observe a sudden spike of salinity? What if frequent rain-after-snow events wash smaller amounts of salt into the environment on a consistent basis? Would the salt aggregate near roads or wash down the river? How are sudden changes in expected weather events affecting the river systems’ ability to buffer salinity? Answering these questions allows us to quantify the resilience of different riverine ecosystems with respect to salt.
With this project, we will analyze riverine data collected across multiple river sites in 15-minute intervals over the course of five years. The objective is to develop models that predict the expected salinity development over time, for a given series of temperature and flow rates. We can query the model to predict how salinity is expected to change with weather patterns by varying the input flow rates and temperatures. We are particularly interested in storm events and other abnormal weather patterns.
In earlier work, the team analyzed both traditional and deep learning models for time series for salinity forecasting. Prediction performance is quantified by ‘root mean squared error’ (RMSE) to the actual salinity. The vast majority of models can accurately predict near futures, but for long horizons deep neural GRU (Gated Recurrent Units) models perform best (3.8 RMSE). However, the accuracy of salinity prediction drops drastically during storm events. With 14.5 RMSE, the best model for storm events is a neural CNN (Convolutional Neural Network), which amounts to a five-fold error-rate increase. Since we are particularly interested in modeling storm events accurately, this performance loss is not acceptable for our purposes.
While we focus on salt concentration in rivers, our algorithms are designed to generalize to other solutes of scientific interest, such as dissolved organic carbon (DOC), nitrogen, and phosphorus. Here we focus on aqueous sensors that are submerged in the river, but our algorithms transfer to sensor data collected in soil and air.
One novel contribution is the “Adjustable Context-aware Transformer model,” which is designed to improve the quality of multi-horizon forecasts over several state-of-the-art methods, including the Transformer model, which has obvious shortcomings in the temporal context. Our model overcomes the challenge that fast dynamics sometimes experience (such as during a rainstorm), where slow processes can dominate. Our trick is, for every time point, to adaptively choose the right context-granularity, which is then used in the forecast. This approach was presented at the American Geophysical Union (AGU) Fall 2021 meeting and published in an ECML European Conference on Machine Learning and Data Mining) workshop on Temporal Data.
A second contribution is a process model to learn the non-linear dependency between covariates (such as flow rate) and target variables (such as salinity). This work is still in progress as of September 2022.
Two students were supported on the seed award: Ph.D. student Sepideh Koohfar (forecasting of solute concentrations) and M.S. student Sarah Hall (process learning of flow-rate solute relationships).
The seed fund project intensified the relationship with researchers in the Natural Sciences, both at our home institution, the University of New Hampshire, and across the United States. Follow-up conversations after our presentation at the American Geophysical Union’s (AGU) Fall 2021 meeting, led to data exchange and a grant proposal that is currently under preparation in 2022.
Several publications have been generated from this Seed Fund research:
Koohfar, S., Wymore, A., McDowell, W., & Dietz, L. (2021, December). “Temporal Context Transformers for Multi-Horizon Prediction of Solute Concentration Responses.” In AGU Fall 2021 Meeting Abstracts (Vol. 2021, pp. H25A-1063).
Sepideh Koohfar & Laura Dietz. (2022). “Adjustable Context-aware Transformer.” 7th Workshop on Advanced Analytics and Learning on Temporal Data. Proceedings of the European Conference on Machine Learning and Principles and Practice of Knowledge Discovery in Databases (ECML).
Sepideh Koohfar (2022). “Adaptive Temporal Pattern Matching.” Women in Machine Learning Workshop at the Thirty-sixth Conference on Neural Information Processing Systems (NeurIPS).
Lead PI: Laura Dietz (University of New Hampshire)
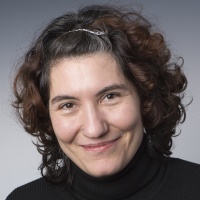
Laura Dietz is an Associate Professor at the department of Computer Science at UNH. Her research focus is text-based machine learning and information retrieval and data science on watersheds.
Previously, she was a Postdoctoral Research Scientist at the Data and Web Science Group of Mannheim University, working with Prof. Simone Paolo Ponzetto. Before that, Dietz was a Research Scientist at the Center for Intelligent Information Retrieval (CIIR), working with Bruce Croft at University of Massachusetts. She also worked as a Postdoctoral Researcher with Andrew McCallum. Dietz graduated from Max Planck Institute for Informatics in Saarbrücken, Germany in January 2011.