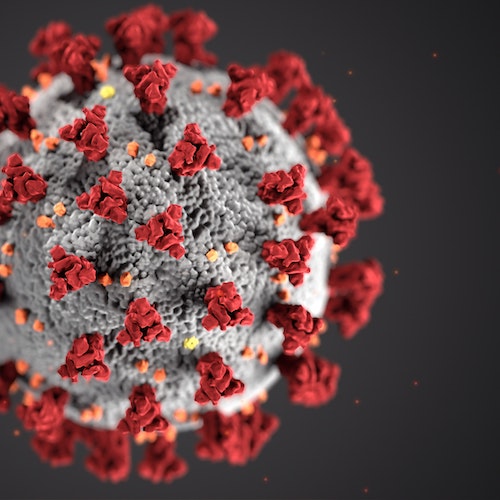
September COVID-19 Research Lightning Talks: Webinar and Q&A
Meet the scientists seeking new insights on COVID-19. Every month, we bring together a group of researchers studying wide-ranging aspects of the current pandemic, to share their research and answer questions from our community. Learn more about their ongoing efforts in the fight against COVID-19, including opportunities for collaboration.
Join us at our next event on Wednesday, September 16, at 2-3pm Eastern Time, featuring lightning talks and Q&A with the following speakers. Register here to receive Zoom information.
_____
Featured Speakers:
Erick Jones, University of Texas at Arlington: EAGER: AI-Enabled Optimization of the COVID-19 Therapeutics Supply Chain to Support Community Public Health. Funded by NSF Engineering / Civil, Mechanical and Manufacturing Innovation.
- This EArly-concept Grant for Exploratory Research (EAGER) will investigate methods that integrate Artificial Intelligence (AI), data science, and automatic data capture technologies to design supply mechanisms that effectively deliver therapeutic medicines to underserved urban communities that are particularly vulnerable to this disease. Learn more about this project here.
Howard Stone, Princeton University: RAPID: Flow Asymmetry in Human Breathing and the Asymptomatic Spreader. Funded by NSF Engineering / Chemical, Biological, Environmental and Transport Systems.
- The focus of this research will be to quantify the complex flows associated with speech and breathing during a conversation or nearby encounter and show how transmission of particles between individuals occurs. The understanding gained from this work will provide actionable mitigation strategies to reduce transmission from asymptomatic people. These outcomes can be useful in the short term by offering routes to reducing potential viral transport and future infections.
Michael Pazzani, University of California San Diego: RAPID: Explainable Machine Learning for Analysis of COVID-19 Chest CT. Funded by NSF Computer and Information Science and Engineering / Information and Intelligent Systems.
- Given the need for rapid, more accurate diagnosis, this project will use, adapt, and evaluate explainable machine learning techniques to diagnosis of COVID-19 pneumonia. This project will improve the understanding of mechanisms of COVID-19 and will help mitigate its impacts.
Ashok Srinivasan, University of West Florida: Collaborative: RAPID: Leveraging New Data Sources to Analyze the Risk of COVID-19 in Crowded Locations. Funded by NSF Computer and Information Science and Engineering / Advanced Cyberinfrastructure.
- The goal of this project is to create a software infrastructure that will help scientists investigate the risk of the spread of COVID-19 and analyze future epidemics in crowded locations using real-time public webcam videos and location based services (LBS) data.
Dominique Duncan, University of Southern California: RAPID: COVID-ARC (COVID-19 Data Archive). Funded by NSF Computer and Information Science and Engineering / Computing and Communication Foundations.
- The goal of this 12-month project is to develop a data archive for multimodal (i.e., demographic information, clinical-outcome reports, imaging scans) and longitudinal data related to COVID-19 and to provide various statistical and analytic tools for researchers.
Debbie Kim, University of Chicago: RAPID: Pandemic Learning Loss in U.S. High Schools: A National Examination of Student Experiences. Funded by NSF Education and Human Resources / Research on Learning in Formal and Informal Settings.
- This project will use two, nationally representative, existing databases of high school students to study their experiences in STEM education during the COVID-19 pandemic. The study is particularly interested in finding patterns of inequities for students in various demographic groups underserved in STEM and who may be most likely to be affected by a hiatus in formal education.
Nora Garza, Laredo College: RAPID: Using real life COVID-19 data to teach quantitative reasoning skills to undergraduate Hispanic STEM students. Funded by NSF Education and Human Resources / Human Resource Development.
- The project at Laredo Community College, a two-year institution, seeks to investigate the effectiveness of using a fully-virtual undergraduate research format to enhance quantitative reasoning and literacy while promoting student persistence during a pandemic of COVID-19. Additionally, this project seeks to promote faculty development in guiding undergraduate research at a community college and in an online learning environment.
Ajitesh Srivastava, University of Southern California: RAPID: ReCOVER: Accurate Predictions and Resource Allocation for COVID-19 Epidemic Response. Funded by NSF Computer and Information Science and Engineering / Advanced Cyberinfrastructure.
- The project will provide a predictive understanding of the spread of the virus by developing machine learning based computational models to study the transmission of the virus and evaluate the impact of various interventions on disease spread. It also formulates and solves a resource allocation problem that can guide the response to contain the epidemic and prevent future outbreaks.